Computer Model Makes Light Work of Telling Faces Apart
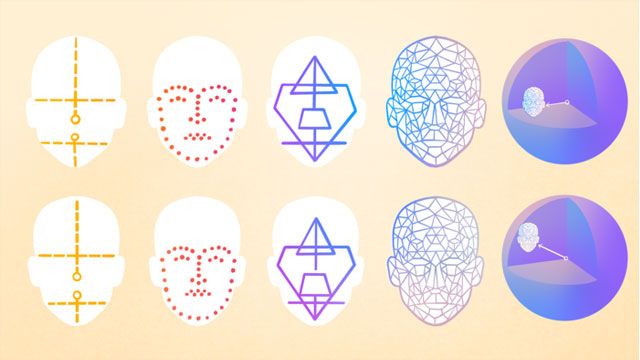
There now are 7.9 billion human faces on the world. All are variations on the identical template: two eyes flanking a nose earlier mentioned a mouth. But with a mere look, most of us can convey to the change involving any two faces. How do our brains make these lightning-quickly judgments?
Spoiler alert: No one appreciates. And though laptop or computer plans right now are great at putting names to faces, none is specially good at judging just how identical (or dissimilar) distinct faces seem. But in a review revealed currently in the Proceedings of the Countrywide Academy of Sciences (PNAS), an worldwide group of researchers screening item-recognition applications report clues about the forms of computations brains may possibly be producing when evaluating the degree of similarity amongst faces.
“Distinguishing faces from a single a different is a pretty incredibly fantastic visual discrimination, nonetheless we are masters at it even when the elements of faces, like noses and cheeks, can look very alike,” said Kamila M. Jozwik, PhD, of the University of Cambridge and the 1st direct author of the paper. “Our research could aid us comprehend how we understand and perceive men and women, which influences how we believe about them and deal with them.”
“The capacity to observe differences even in the exact same deal with, as in facial expressions or in common faces that have aged, influences our emotions and how we interact with persons,” added Katherine R. Storrs, PhD, of the Justus Liebig College Giessen, Germany, and the next lead author of the review.
In their paper, Drs. Jozwik and Storrs, Nikolaus Kriegeskorte, PhD, a principal investigator at Columbia’s Zuckerman Institute and senior creator of the examine, and many coauthors, identified a “surprisingly simple” laptop or computer product that proved to be very good at gauging facial variances.
“We hope this offers us with theoretical insight into the computational approach our brains make when recognizing familiar faces or encountering new ones,” stated Dr. Kriegeskorte.
Toward that conclusion, the researchers recruited 26 undergraduates at the University of Cambridge and asked them to rank many pairs of practical computer-generated faces (based on scans of genuine faces) with respect to how equivalent the two faces in every single pair appeared.
The scientists then tasked 16 diverse laptop or computer applications, each and every functioning a model that represented faces in a distinctive way, to make the exact same facial area similarity rankings. Some of the styles represented faces as digital pictures, substantial preparations of pixels. Some versions relied on geometric meshes, whose facets can be altered to stand for faces. However some others produced comparisons of facial landmarks. Last but not least, some versions applied details about textures and the styles of attributes this sort of as eyebrows, or had been by themselves based mostly on artificial intelligence devices.
“We were looking for a personal computer design that would make the exact same judgments individuals do when comparing faces,” reported Dr. Jozwik. “That would set us in a fantastic setting up position to inquire how the mind compares unique faces.”
The scientists observed that two varieties of models were being most effective at replicating the students’ similarity rankings. One kind, deep neural networks (DNNs), is used on our mobile phones to realize faces in pics and is usually depicted in flicks and Tv set demonstrates whose storylines contain AI.
Programmers educate DNNs to figure out an object, say, a cat or a human encounter, with galleries of electronic photos that have earlier been annotated by a man or woman as getting examples of the item of desire. The education section consistently readjusts the sequence of calculations that DNNs make until these synthetic intelligence applications can spot the goal object in new photos introduced to the designs. In the examine, the DNN designs in comparison the many thousands of pixels comprising distinctive faces. From all those comparisons, the DNNs calculated similarity rankings in between the exact same deal with pairs the pupils rated.
The other kind of plan that was in particular superior at replicating the student’s facial similarity judgements was derived from the Basel Deal with Product (BFM). Believe of the BFM as a type of electronic clay for faces. By massaging many portions of digital faces (scanned from authentic persons), it gets to be doable to morph a encounter, more or fewer subtly, into a various experience and shapes and textures can be specifically and mathematically specified. For the PNAS examine, researchers developed pairs of faces from this BFM model and questioned pupils to arrange them on a huge personal computer contact display screen in accordance to how similar the facial area pairs seem.
The most placing final result, the researchers said, is that the BFM was as fantastic as the considerably additional computationally intense DNN models in replicating the facial-similarity perceptions of the pupils. This outcome implies that the types of statistical versions among faces assessed by the BFM product are critical to our brains, stated Dr. Storrs.
The scientists anxiety that their research has limitations. For 1 point, the BFM was developed by scientists in Basel, Switzerland, centered on scans of 200 typically youthful, White faces. “The organic variation in a populace of faces is distinctive for different individuals in distinctive locations,” explained Dr. Kriegeskorte. Unavailable at the second are equipment and datasets that are consultant of the world’s facial variety. That restrictions the self-confidence the scientists can at present have that their get the job done does, in reality, issue toward the brain’s own computational tactics for assessing faces.
“Our hope is that these results can guideline us towards analysis issues and methods that will unveil more exactly in which and how in the brain this critical information and facts-processing undertaking is likely on,” said Dr. Kriegeskorte. “We also hope investigation like ours will assistance us have an understanding of the inner workings and shortcomings of artificial intelligence devices for recognizing faces, which are getting a lot more widespread in our technological landscapes.”
Reference: Jozwik KM, O’Keeffe J, Storrs KR, Guo W, Golan T, Kriegeskorte N. Face dissimilarity judgments are predicted by representational distance in morphable and image-computable versions. PNAS. 2022119(27):e2115047119. doi: 10.1073/pnas.2115047119
This posting has been republished from the pursuing products. Notice: materials may well have been edited for length and content. For further data, be sure to get in touch with the cited resource.